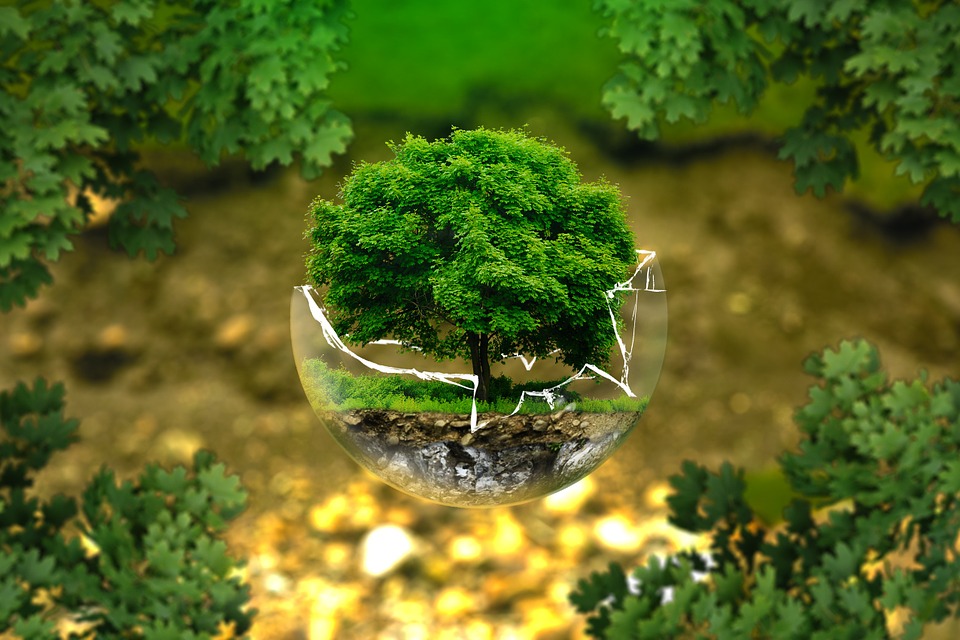
Reproducibility in Ecology
1 Introduction to the Reproducibility Crisis
The replication crisis is believed to be an ongoing crisis of methodology in science. It is based on the observation that a large number of scientific studies across multiple fields have been found to be difficult or even impossible to replicate or reproduce. [1] As people of science in the 21st century where everyone is a master of photo editing apps and unproven hypotheses are used to spread misinformation using social media, it is almost sure that we will have to face the implications that this “crisis” brings with it. Some of us might already have seen the face of this struggle. Sometimes, even in the most deterministic of subjects like physics or computational neuroscience, this struggle leaves one baffled when even simple results from one’s own lab cannot be replicated. There have been some historical cases where the outcomes of this crisis came to the front of the world such as Hideyo Noguchi’s Syphilis Bacteria, University of Utah “Cold Fusion” Experiment, Telsa’s Wireless Transmitter, MMR vaccine controversy (vaccine caused autism) - a historical case of fraud, the Faster-Than-Light Neutrinos and others.
The terms we use for this, i.e., “reproducibility crisis” and “replication crisis” has gained momentum in print and conversation only over the last decade [2], but this crisis has its roots in what we consider science itself and the problem lies in the way we do science. Karl Popper, one of the people responsible for our current philosophy of science, proposed repeatability as a critical tenet of what is correct in science. He said, “non-reproducible single occurrences are of no significance to science.” [3] Ronald Fisher, the man behind Hypothesis Testing- the foundation of modern science, said that “we may say that a phenomenon is experimentally demonstrable when we know how to conduct an experiment which will rarely fail to give us statistically significant results.” [4] Thus, if the data cannot be reproduced, well, it is not science.
The issues existed but they only came to notice recently as disappointing results emerged from large scale reproducibility projects in various medical, life, and behavioral sciences such as the Open Science Collaboration (2015) [5] and a recent survey by Nature (2016) [6] showed that 52% of over 1500 surveyed scientists agree that there is a severe reproducibility crisis and another 38% agreeing that there is such a crisis. The problem of reproducibility is neither a recent problem nor a dismissable one. There is an almost complete absence of studies aimed at validating and replicating published literature in many scientific fields despite the widespread failure to reproduce results. Up to 80% of Biologists and Chemists claimed in Nature 2016 survey [6] that they could not replicate someone else’s results, and 60% could not even replicate their own results. It has been shown that there is also a very high prevalence of questionable research practices and publication bias where most seemingly "unimportant" negative results go unpublished, artificially increasing the rate of false positives in the literature. [7] There is also the problem of the lack of clarity and completeness in the reporting of methods, raw data, and data analysis. The prevalence of these issues of the way we do science has been well documented. [8]
The Nature (2016) survey [6] asked the question of what might be causing this crisis. Most of the scientists (more than 60%) believed that two major factors contribute to the reproducibility crisis - the pressure to publish and the process of selective reporting to suit one’s hypothesis. More than half of the respondents think that these factors were also supplemented by other issues poor oversight and lack of checks against malpractice, insufficient replication attempts in the lab, or low statistical power. In 1977, Michael Mahoney showed confirmation bias thrives in peer review “Confirmatory bias is the tendency to emphasize and believe experiences which support one’s views and to ignore or discredit those which do not...reviewers were strongly biased against manuscripts which reported results contrary to their theoretical perspective.” [9] Besides these reasons, few people also blamed the unavailability of code, specialized methods, and raw data, variability in reagents used, bad experiment design, fraud, insufficient peer review and some also suggested lousy luck to be a cause of the reproducibility crisis. [6]
Nevertheless, how do we address this problem? More than 50% of the scientists in the Nature survey agreed to some steps we could take: Better Understanding of Statistics, Better Mentoring, More robust Experimental Design, Better Teaching, More within-lab validation, Incentives for better practice, Incentives for publishing reproductions, More external-lab validation, Journal Enforcing Standards (in order of preference.) [6] There have already been a few steps in the right direction, but we are still a long way from the ideal world of replicable, reproducible sciences. Formal frameworks have been established for meta-analysis of clinical results and research articles. In 2016, the American Statistical Association (ASA) played its part and issued its first-ever guidelines in an attempt to stem the rising tide of misuse and misinterpretation of p-values in the field of research. [10] Many countries and institutions have started their projects to support the replication of the existing body of literature in various fields. With the “reproducibility crisis” on our hands, we need to try our best to prevent unwanted biases in the way we design, conduct, interpret, and report experiments and at the same time question what we already know and try to replicate it, no matter how hard it is. However, ‘science” is not scientific without all of that. [11]
2 Reproducibility Crisis in Ecology
Now that we have discussed the crisis of “reproducibility” in science let us focus on one particular field, the field of ecology and evolutionary sciences. As per the methodology and philosophy of science, the field of Ecology has often been compared to the Social Sciences, both of which have seldom been tagged as Soft Sciences. [12] In the plethora of scientific fields, the field of Psychology is most plagued by the problems of reproducibility, and so it is not a surprise that Ecology too faces a lot of similar issues in making replicable and reproducible claims. [13] Ecology heavily relies on statistics, and as mentioned earlier, due to the problems of non-robust statistics and low statistical power, science is currently also facing a “Statistical Crisis” where statistically “significant” results do not hold up to reality. [14] People often are subject to the charm of questionable research practices (QRPs) to suit their hypotheses. [15] Rather than an unbiased analysis of the raw data, datasets are collected, keeping in mind the hypothesis which they desperately want to be accurate, so that they can get published in a “High Impact Factor” Journal. This leads to what one might call “cherry-picking data”.
There was an article published in PLoS One (2018) on the prevalence of QRPs in the field of Ecology. [15] A team of scientists (or one might lovingly call them meta-scientists) led by Hannah Fraser from the University of Melbourne surveyed around 500 ecologists and 300 evolutionary biologists. A primary cause of psychology’s replication crisis was that researchers groomed raw data to make it look more appealing and closer to the results predicted. Shockingly, the survey found that 64% of surveyed researchers had at least once failed to report results because they were not statistically significant (a clear case of cherry-picking). Around 42% had collected more data after inspecting whether results were statistically significant, which is a well-known and infamous form of what is called p-hacking. P-hacking is the use of data mining to uncover apparent (or even possibly real) correlations that were not included in the original hypothesis and artificially inflating the significance via transformation not based on logic. A well-known consequence of p-hacking is that “nonsignificant results become significant”. [16] Moreover, 51 % reported an unexpected finding as if it had been the expected hypothesis from the start itself, a process known colloquially as HARKing. These malpractices have been directly linked to the low rates of reproducible results in psychology and other disciplines. Thus, a drive and incentive to ensure statistical honesty, and education and unbiased analysis can help alleviate the crisis. [16,17]
Fidler (2017) argues that in a few cases, the “direct replication” of research in ecology is due to strong spatial and temporal dependencies and proposes “meta-research projects” to provide indicative measures of reproducibility such as “transferability”. [18] Transferability is a form of inferential reproducibility where the raw data itself is not reproducible, but the general principle it predicts or the inference it makes is the same. A few recent studies in ecology have addressed this issue, such as Yates (2018) "Review on Transferability " [19], Kleiven (2018) "Seasonal Differences in an Ecological Model" [20], Soininen (2018) "Temporal Transferability in Plant Rodent Interactions" [21].
Another major problem that Ecology faces is that distinction is often not made between reproducibility among experiments and reproducibility within the same experiment. [22] Ecology has historicity and a large number of factors that affect the results. [12] Experiments conducted at slightly different times on different systems or with even slightly different methods can give dramatically different results. [12] Reproducibility within the same experiment can always be achievable by increasing sample sizes. Ives concludes in his article titled “Informative Irreproducibility and the Use of Experiments in Ecology” in BioSciences (2018) [22] that experiments in ecology must face judgment on the grounds of what they reveal to us about the “system under study” in “a strict statistical way.”
Furthermore, the judgment should be on whether the questions are “ecologically interesting” and may contribute “qualitative insights into other systems.“ They definitely should not be judged on the reproducibility and “quantitative statistical comparisons among experiments,” primarily if the studies do not aim to make such a claim. [22] One must admit that ecology has many factors, all of which cannot always be controlled for, Context Matters. The knowledge we gain from differences in research outcome should be ascribed to changes in context, rather than a failure to replicate an earlier study. One must settle for a middle ground, a point where the collection and analysis are robust, not cherry-picked, and repeatable for the given conditions but may not be reproducible at another’s hand because even such a result can direct us to general principles. All one has to do after that is a sleuths’ work of finding the real culprit (the general principle). [12]
Thus in the face of all these issues, we must remember a few things. As Schloss (2018) [23] puts it, at any point, we should consider as many factors as possible and avoid attributing issues with reproducibility, replicability, robustness, and generalizability to “a dim view of our fellow scientists as being sloppy, biased, or untrustworthy.” Beyond correctness, the aim should be to create work that future scientists can build upon. Moreover, remember there is much room for improvement, but we have to acknowledge that “science is a process of learning and that it is really freaking hard.”
References:
[1] Peng, R. The reproducibility crisis in science: A statistical counterattack. Significance, 12: 30-32, (2015).
[2] Pashler, Harold, and Eric–Jan Wagenmakers. "Editors’ introduction to the special section on replicability in psychological science: A crisis of confidence?." Perspectives on Psychological Science 7.6 (2012): 528-530.
[3] Popper, Karl. The logic of scientific discovery. Routledge, 2005.
[4] Fisher, Ronald A. "The design of experiments." (1949).
[5] Open Science Collaboration. "Estimating the reproducibility of psychological science." Science 349.6251 (2015): aac4716.
[6] Baker, Monya. "1,500 scientists lift the lid on reproducibility." Nature News 533.7604 (2016): 452.
[7] Fidler, Fiona, and John Wilcox. "Reproducibility of scientific results." (2018).
[8] Nuijten, Michèle B., et al. "The prevalence of statistical reporting errors in psychology (1985–2013)." Behavior research methods 48.4 (2016): 1205-1226.
[9] Mahoney, Michael J. "Publication prejudices: An experimental study of confirmatory bias in the peer review system." Cognitive therapy and research 1.2 (1977): 161-175.
[10] Wasserstein, Ronald L., and Nicole A. Lazar. "The ASA’s statement on p-values: context, process, and purpose." The American Statistician 70.2 (2016): 129-133.
[11] Bastian, Hilda. "Reproducibility Crisis Timeline: Milestones in Tackling Research Reliability", Absolutely Maybe, Evidence and uncertainties about medicine and life. PLoS Blogs, 5 Dec. 2016. Web. 25 Oct. 2019.
[12] Pigliucci, Massimo. "Are ecology and evolutionary biology" soft" sciences?." Annales Zoologici Fennici. Finnish Zoological and Botanical Publishing Board, 2002.
[13] Yoccoz, Nigel Gilles. "The replication and reproducibility crises: origins and consequences for studies of ecology and evolution." Septentrio Conference Series. No. 1. 2018.
[14] Gelman, Andrew, and Eric Loken. "The statistical crisis in science." The best writing on mathematics 2015 (2016): 305.
[15] Fraser, Hannah, et al. "Questionable research practices in ecology and evolution." PloS one 13.7 (2018): e0200303.
[16] Head, Megan L., et al. "The extent and consequences of p-hacking in science." PLoS biology 13.3 (2015): e1002106.
[17] Fisher, Ronald Aylmer. "Statistical methods for research workers." Breakthroughs in statistics. Springer, New York, NY, 1992. 66-70.
[18] Fidler, Fiona, et al. "Metaresearch for evaluating reproducibility in ecology and evolution." BioScience 67.3 (2017): 282-289.
[19] Yates, Katherine L., et al. "Outstanding challenges in the transferability of ecological models." Trends in Ecology & Evolution 33.10 (2018): 790-802.
[20] Kleiven, Eivind Flittie, et al. "Seasonal difference in temporal transferability of an ecological model: near-term predictions of lemming outbreak abundances." Scientific reports 8.1 (2018): 15252.
[21] Soininen, Eeva M., et al. "Transferability of biotic interactions: Temporal consistency of arctic plant–rodent relationships is poor." Ecology and Evolution 8.19 (2018): 9697-9711.
[22] Ives, Anthony R. "Informative Irreproducibility and the Use of Experiments in Ecology." BioScience 68.10 (2018): 746-747.
[23] Schloss, Patrick D. "Identifying and overcoming threats to reproducibility, replicability, robustness, and generalizability in microbiome research." MBio 9.3 (2018): e00525-18.